The Role of Ethical AI in Healthcare: Strategies & Real-World Examples
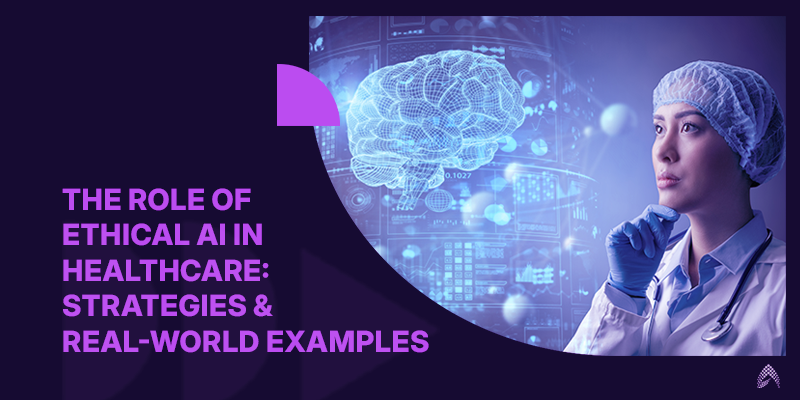
Ethical AI In Healthcare Industry
In 2019, a widely used AI system designed to help hospitals allocate healthcare resources was exposed for favoring white patients over black patients, despite both having the same level of medical need. The bias wasn’t intentional—rather, it stemmed from flawed training data that used historical healthcare spending as a proxy for patient needs. Since Black patients had historically less access to healthcare, their spending was lower, causing the AI to deprioritize their care. This wasn’t just an ethical AI failure—it was a systemic risk that reinforced existing health disparities.
This case isn’t an anomaly. AI models across diagnostics, treatment recommendations, and administrative decision-making are prone to bias, lack of transparency, and privacy risks. Without ethical safeguards, AI can exacerbate inequalities rather than eliminate them.Â
This guide explores why ethical AI in healthcare matters, the risks of neglecting it, and a practical framework to ensure AI is trustworthy, fair, and patient-centered. The future of healthcare AI isn’t just about innovation—it’s about ensuring that innovation serves all patients equitably.
Key Takeaways:
​​Healthcare AI systems can perpetuate or worsen health disparities when implemented without ethical safeguards. A 2019 case study revealed AI bias in resource allocation favoring white patients due to historical spending data.
Data privacy breaches in healthcare are escalating dramatically, with a 192% increase in 2023 and affecting 82% of the US population by 2024, highlighting the critical need for robust security measures.
Successful ethical AI implementation in healthcare requires a multi-stakeholder approach. The Mayo Clinic demonstrated this with their explainable AI (XAI) in radiology, improving both diagnostic accuracy and physician trust.
Organizations must measure ethical AI in healthcare performance through specific KPIs, including fairness scores, bias detection rates, and transparency indices. Case studies show AI dermatology tools can be up to 40% less accurate for darker skin tones without proper diversity in training data.
Privacy-first techniques like federated learning and encrypted data processing are becoming essential, not optional, for healthcare AI implementation. This ensures HIPAA and GDPR compliance while maintaining AI effectiveness.
Building trust requires transparent communication with patients about ethical AI use in their care. Healthcare providers should implement clear consent processes and patient education initiatives to improve AI literacy and engagement.
Why Ethical AI in Healthcare Matters
AI has the potential to enhance diagnostic precision, create tailored treatment plans, improve patient financial experiences, and streamline hospital workflows. However, these advancements mean nothing if patients and healthcare providers don’t trust AI to be fair, transparent, and secure.
A lack of transparency in AI-driven decision-making can make it difficult for doctors and patients to understand why specific medical recommendations are made. Moreover, AI bias in healthcare concerns is rising, as AI requires vast amounts of personal health data to function effectively.
This lack of confidence highlights the urgent need for ethical AI frameworks that prioritize fairness, transparency, and accountability. If healthcare organizations fail to address these concerns, Artificial Intelligence in healthcare could face resistance, limiting its ability to improve patient outcomes and reduce healthcare disparities.
Ethical AI as a Competitive Advantage
AI is transforming healthcare, enhancing diagnostic accuracy, optimizing treatment plans, and streamlining operations. However, the difference between unethical and ethical AI in healthcare can mean the difference between life-saving care and harmful misdiagnoses. A well-designed, ethical AI system can improve patient outcomes and build trust, while a biased or opaque AI model can exacerbate health disparities, misallocate resources, and erode confidence in healthcare technology.
Healthcare AI: Ethical AI in Action
The Mayo Clinic provides a prime example of how ethical AI can improve patient care. They implemented explainable AI (XAI) models in radiology, allowing doctors to see how AI reached its conclusions when analyzing medical images. This transparency helped radiologists validate AI recommendations, detect errors, and confidently incorporate AI into decision-making. The result? Higher diagnostic accuracy, faster detection of abnormalities, and improved patient outcomes.Â
The Consequences of Unethical AI
Compare this to an AI-driven dermatology tool that was found to be 40% less accurate for patients with darker skin tones. The failure occurred because the AI model was primarily trained on light-skinned patients, meaning it struggled to detect skin conditions like melanoma in patients with darker skin. This lack of diverse data led to higher rates of misdiagnosis, putting lives at risk. Patients of color were left vulnerable, highlighting why fairness and diversity in AI training data are non-negotiable.
Learn more about the evolution of AI in 2025.
Without Ethical AI: Challenges Businesses Can't Avoid
AI implementation in healthcare without a strong ethical framework can lead to several issues:
Informed Consent Issues
Patients may be unaware of how their data is used in AI applications, leading to a lack of informed consent. It's essential for healthcare providers to ensure patients understand and agree to the use of their data in AI systems.
Lack of Transparency
AI systems often operate as "black boxes," making it difficult for healthcare providers and patients to understand how decisions are made. This opacity can lead to mistrust and reluctance to adopt AI-driven solutions.
Algorithmic Bias and Fairness Gaps
If AI models are trained on biased data, they can perpetuate or even exacerbate existing health disparities. For example, an AI system trained predominantly on data from one demographic may not perform well for others, leading to unequal care.
Data Privacy Risks
Poorly secured AI systems are vulnerable to cyberattacks, unauthorized access, and data leaks, putting patients’ sensitive medical histories at risk. Organizations that fail to prioritize strong encryption, data anonymization, and regulatory compliance not only endanger patient privacy but also face hefty legal fines and reputational damage.
Regulatory Non-Compliance Risks
Failing to adhere to ethical ai standards can result in legal penalties, reputational damage, and loss of patient trust. Regulatory bodies are increasingly scrutinizing AI applications in healthcare to ensure they meet ethical and legal standards.
In 2021, 60 million healthcare records were breached, and 57 million in 2022, but the following year saw a 192% increase to 168 million breached records, followed by a 63.5% increase to 275 million records in 2024. Last year, the records of 82% of the population of the United States were exposed, stolen, or impermissibly disclosed.
How to Implement Ethical AI In Healthcare: Guide
Implementing AI ethics in healthcare is not just about regulatory compliance—it’s about building trust, ensuring fairness, and prioritizing patient safety. For organizations looking to integrate AI responsibly, the following actionable steps provide a structured approach to governance, transparency, fairness, privacy, and explainability.
1. Build a Strong AI Governance Framework
What to Do:
Establish an AI ethics committee that includes clinicians, ethicists, AI engineers, legal experts, and patient advocates.
Define clear accountability structures to determine who oversees AI decision-making and system outcomes.
Align governance policies with global regulations such as HIPAA, GDPR, and the FDA’s AI guidelines.
Conduct regular AI ethics audits to assess compliance, fairness, and potential risks.
2. Ensure Informed Consent and Data Transparency
What to Do:
Create clear, accessible AI consent forms that explain:
- Why AI is used in diagnosis or treatment.
- What patient data is collected and how it is processed.
- Potential risks and benefits of AI-driven decisions.
Implement patient education initiatives to improve AI literacy and encourage informed decision-making.
Develop real-time patient data access portals, allowing individuals to track how their health data is used in AI models.
3. Mitigate Bias Through Fair AI Training
What to Do:
Use diverse, representative datasets that include patients from different ethnic, gender, and socioeconomic backgrounds.
Implement bias detection tools like IBM AI Fairness 360 or Google’s What-If Tool to identify and correct disparities.
Conduct fairness audits before deploying AI in real-world clinical settings.
Train AI models on inclusive medical literature and datasets to reduce diagnostic disparities.
4. Strengthen Data Privacy and Security
What to Do:
Adopt privacy-first AI techniques, such as federated learning, to process patient data without centralizing sensitive information.
Encrypt all patient data and implement multi-layer access controls to protect against breaches.
Conduct regular cybersecurity audits to assess vulnerabilities in AI-driven systems.
Comply with HIPAA, GDPR, and local data protection laws to ensure responsible AI deployment.
5. Prioritize Explainability and Transparency
What to Do:
Develop explainable AI (XAI) models that provide clear insights into AI-driven decisions.
Train healthcare professionals on how to interpret AI-generated recommendations before integrating them into clinical workflows.
Use visual dashboards and interactive tools to make AI predictions more transparent to both clinicians and patients.
Encourage human oversight, ensuring AI assists, rather than replaces, medical decision-making.
How to Measure Ethical AI Implementation: Key Performance Indicators (KPIs)
Organizations must establish measurable KPIs to monitor fairness, transparency, and accountability to ensure ethical AI practices.
Fairness Score
Measures whether AI models provide equitable treatment across different demographic groups.
Â
How to Track It:
- Conduct demographic performance audits on AI predictions.
- Use bias detection tools like Google’s What-If Tool or IBM AI Fairness 360.
- Compare AI-generated diagnoses against historical patient outcomes.
Bias Detection Rate
Tracks how frequently AI requires bias-related adjustments post-deployment.
Â
How to Track It:
- Run bias audits every 6-12 months to detect shifts in AI accuracy across patient groups.
- Compare misdiagnosis rates before and after AI interventions.
- Implement real-time AI fairness monitoring dashboards.
Transparency Index
Assesses the explainability of AI outputs for medical professionals and patients.
Â
How to Track It:
- Survey clinicians on AI interpretability in decision-making.
- Assess how often doctors override AI-generated recommendations due to lack of clarity.
- Deploy Explainable AI (XAI) tools that provide decision rationales in medical reports.
Patient Consent Compliance Rate
Evaluates whether AI systems obtain proper informed consent before processing sensitive data.
Â
How to Track It:
- Track the percentage of AI-powered treatments or diagnostics that include patient-approved consent forms.
- Implement digital consent platforms that allow patients to review and approve AI interactions.
- Conduct annual compliance audits to ensure adherence to HIPAA, GDPR, and local regulations.
Data Privacy Compliance Score
 Ensures AI adheres to HIPAA, GDPR, and other relevant healthcare regulations.
Â
How to Track It:
- Conduct quarterly cybersecurity audits to detect vulnerabilities.
- Measure compliance with data encryption, anonymization, and access control policies.
- Monitor incident reports on unauthorized AI-driven data access.
Human-AI Collaboration Metrics
Examines the impact of AI on clinical decision-making and patient outcomes.
How to Track It:
- Analyze whether AI recommendations align with or enhance clinician decisions.
- Measure time saved per diagnosis and reduction in misdiagnosis rates.
- Track physician adoption rates of AI-assisted tools in clinical workflows.
Healthcare organizations can ensure that their AI technologies align with long-term ethical standards by establishing real-time monitoring systems and conducting routine AI ethics audits.
Conclusion
As AI and healthcare accelerates, organizations must ensure ethical implementation is at the core of their strategy. How is your institution addressing issues of ethical AI in healthcare? Start by auditing your current AI models for bias, transparency, and compliance today.
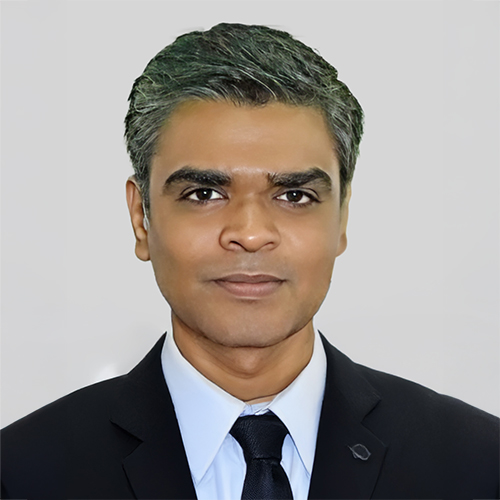
Director ATG & AI Practice