AI in 2025: Realistic Timelines, Challenges, and Measurable Success
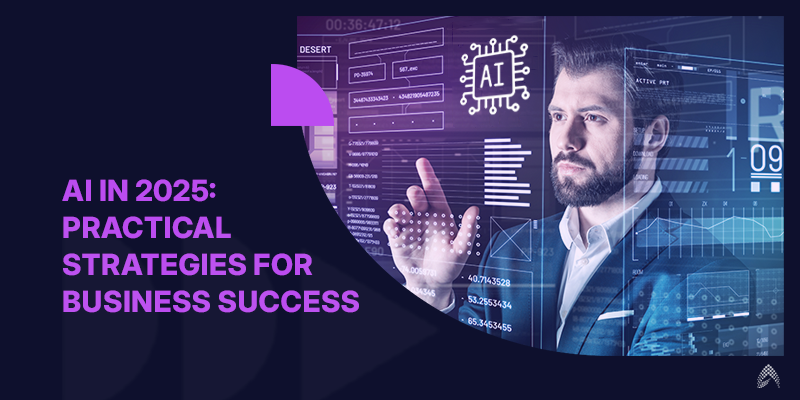
While headlines scream about AI taking over the world, the reality on the ground is far more nuanced. The hype surrounding artificial intelligence can be deafening, painting a picture of overnight transformation across all industries. However, a more practical perspective reveals a different story: the true evolution of AI will be a gradual, iterative process, driven by practical implementation and measurable results. This post explores a more realistic timeline for AI adoption, focusing on actionable steps, persistent challenges, and the significance of measuring tangible success.
The Myth of Overnight AI Transformation
The notion that businesses can flip a switch and instantly become AI-powered is a widespread misconception. While the theoretical capabilities of AI are undoubtedly impressive, translating them into real-world applications is a different ballgame. Integrating AI into existing systems, workflows, and organizational structures requires extensive effort, investment, and expertise. Many businesses are still wrestling with fundamental data management challenges, a critical foundation for effective AI deployment.Â
According to a 2023 Gartner report, 80% of AI projects fail or remain prototypes due to data challenges and integration issues. This disparity between AI’s potential and its practical realities underscores the need for a phased, incremental approach rather than aiming for a total transformation overnight. Step-by-step improvements offer the most viable path toward meaningful AI adoption.
Real Adoption Timelines: A Pragmatic Outlook
Instead of predicting AI dominance in every facet of life by 2025, it’s more practical to focus on areas where adoption is genuinely gaining momentum:
Automation of repetitive tasks
Robotic process automation (RPA) is streamlining back-office operations, while AI-powered chatbots are efficiently managing routine customer inquiries. These technologies will continue to evolve, handling increasingly complex and rule-based processes. For example, in the healthcare industry, AI is automating administrative tasks like patient intake and appointment scheduling, freeing up valuable time for medical staff to focus on direct patient care.
Data analysis and insights
AI analytics tools are revolutionizing how businesses extract value from massive datasets, enabling smarter decision-making in areas such as marketing, operations, and supply chain management. In retail and finance, AI-driven platforms are helping companies forecast demand, optimize inventory, and predict consumer behavior, driving efficiency and reducing costs.
Personalized experiences
AI-driven recommendation engines and marketing platforms are offering hyper-personalized user experiences, anticipating customer needs with greater precision. For instance, e-commerce companies are leveraging AI to recommend products based on customer browsing habits, significantly improving conversion rates and customer loyalty.
The pace of adoption depends on factors such as the availability of quality data, implementation costs, access to skilled professionals, and regulatory frameworks. Recognizing these realities, transformative AI adoption will unfold as a series of progressive milestones rather than a sudden, all-encompassing revolution.
Implementation Realities: Navigating the Hurdles
Successfully implementing AI comes with its fair share of challenges, including:
Data quality and availability
Poor data quality often derails AI projects, as AI systems rely heavily on clean, structured data to generate meaningful outputs. The "garbage in, garbage out" adage holds particularly true here.
A study by MIT Technology Review Insights found that more than 53% of respondents cite data quality issues as the top barrier to AI adoption.
Integration with existing systems
Retrofitting AI into legacy systems is complex and requires careful planning, technical expertise, and substantial resources.
Talent acquisition and training
The shortage of skilled AI professionals makes it difficult for businesses to build and retain the necessary expertise in-house.
Change management
Adapting workflows and processes to integrate AI often faces resistance from employees accustomed to traditional methods.
Security and privacy concerns
Protecting sensitive data used in AI systems is crucial, especially in regulated industries like healthcare and finance.
To overcome these hurdles, organizations should start with targeted pilot projects that solve specific business problems, prioritize data quality, and foster cross-departmental collaboration to ensure holistic success.
Defining and Measuring Success: Moving Beyond the Hype
Vague goals like “enhancing efficiency” or “improving customer experience” fall short when assessing AI’s impact. Instead, businesses must establish measurable key performance indicators (KPIs) tied to tangible outcomes, such as:
Cost reduction
Track savings in operational expenses like labor and material costs.
Increased productivity
Measure improvements in efficiency, throughput, and resource utilization.
Improved accuracy
Monitor reductions in errors, defects, and inconsistencies in processes.
Enhanced customer engagement
Evaluate metrics like retention rates, satisfaction scores, and conversion rates.
For instance, rather than a vague goal of “enhanced customer satisfaction,” a concrete target like “achieving a 10% increase in customer retention within six months” provides a clear benchmark for success.
Practical Steps for AI Adoption
Businesses embarking on their AI journey can follow these logical steps:
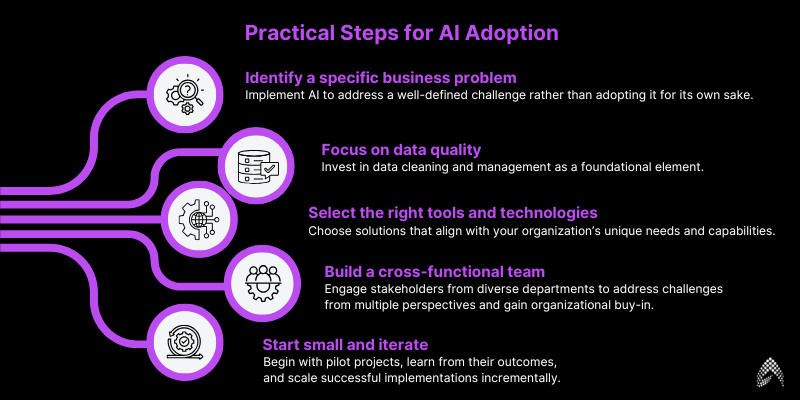
Implement AI to address a well-defined challenge rather than adopting it for its own sake.
Invest in data cleaning and management as a foundational element.
Choose solutions that align with your organization’s unique needs and capabilities.
Engage stakeholders from diverse departments to address challenges from multiple perspectives and gain organizational buy-in.
Begin with pilot projects, learn from their outcomes, and scale successful implementations incrementally.
Embracing the AI Revolution
AI’s potential to transform industries is undeniable, but achieving its full benefits requires a more rational mindset, realistic timelines, and a focus on practical implementation. The real AI transformation lies in steady, incremental progress aimed at solving genuine business problems and delivering measurable outcomes.
Instead of succumbing to the hype, organizations should develop clear strategies aligned with their business goals. Understanding the data landscape, identifying opportunities for value creation, and crafting an actionable roadmap are critical steps toward successful AI adoption. Recognizing AI as a business and organizational challenge—not merely a technological one—further emphasizes the importance of adaptability, collaboration, and continuous learning.
Partnering for AI Success
Navigating AI’s complexities often requires expert guidance. From data preparation to deployment, the journey involves numerous technical and strategic considerations. Partnering with a trusted advisor can provide invaluable support. At Amzur, we help businesses overcome these challenges by offering tailored AI solutions that address specific needs. By emphasizing real-world applications, collaborative problem-solving, and measurable results, we enable our clients to unlock AI’s transformative potential
We helped a California-based HVAC servicing company automate their technician assignment process. By developing an AI-powered recommendation engine, we reduced technician assignment time by 50%, improving operational efficiency and customer satisfaction. This highlights the power of AI in driving tangible improvements across industries.
If your organization is looking to embark on an AI journey or enhance existing initiatives, we’re here to guide you every step of the way.
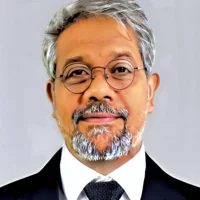
President & CEO – Growth Markets