How to Scale AI in Healthcare: Strategies & Future
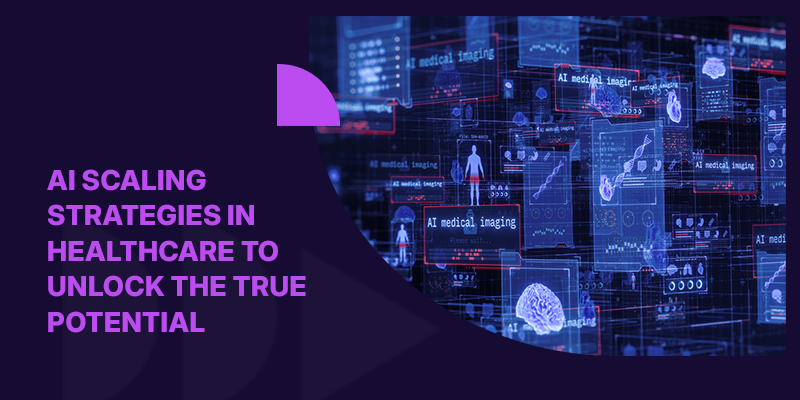
AI Scaling Strategies In Healthcare To Unlock the True Potential
AI is evolving rapidly, with Generative AI (GenAI) models being released every few days, opening up new possibilities for industries worldwide. Despite the advancements, CIOs often report that AI hasn’t yet met ROI expectations—a challenge that healthcare and other sectors are grappling with as they navigate the AI landscape. The key to overcoming this challenge lies in adopting AI scaling strategies efficiently and ensuring its implementation is not only ambitious but also strategically sound.
In 2024, 74% of CEOs believe AI will transform their industries, underscoring the importance of getting AI implementation right. With the right scalable AI strategy, businesses can unlock the true potential of AI—driving innovative solutions, increased efficiency, and measurable ROI. [Gartner Report on AI success and scaling]
Importance of Scaling AI in Healthcare: A Simple Example from Radiology
In the radiology department, scalable AI strategies can revolutionize how healthcare professionals diagnose and treat patients. AI reduces CT lung cancer screening workload by nearly 80% [Source] is a game-changer for radiology departments, and it highlights the profound potential of AI in healthcare. Lung cancer screening, particularly through CT scans, is a critical diagnostic tool used to identify cancer in its early stages, significantly improving the chances of successful treatment.Â
AI tools equipped with deep learning algorithms can automatically segment images, detect abnormalities, and flag potential signs of lung cancer. By automating 80% of the workload, AI in Radiology operations enables radiologists to focus on the most complex cases, reducing stress, fatigue, and ultimately, the risk of diagnostic errors due to human oversight.
AI scaling across the entire radiology department, and even multiple healthcare sites, ensures that the diagnostic process remains consistent, regardless of the radiologist’s experience level or the location of the screening.Â
AI Scaling strategies in healthcare can ensure standardization of the interpretation of CT lung cancer scans across different facilities, reducing variability and ensuring that every patient receives the same level of care.
This AI scalability enables hospitals and healthcare organizations to expand their services, allowing radiologists to maintain high standards of care and accuracy without being overwhelmed by growing patient volumes.
Challenges in Scaling AI Projects:
AI scaling in healthcare presents several formidable challenges that hinder its widespread adoption and effective implementation. Addressing these obstacles is crucial for harnessing AI’s potential to enhance patient outcomes and streamline healthcare operations. Here are seven key challenges:​
1. Data-Related Challenges
Challenges:
Data Quality
AI project’s success depends on the quality of data it is processing. However, inaccurate, inconsistent, or incomplete data results in poor model performance.
Data Availability & Siloes
Data Governance & Security
2. Technical Complexity
Challenges:
Integration
Healthcare organizations operate a multitude of legacy systems that may not be compatible with new AI technologies. Integrating AI solutions into these existing infrastructures requires significant technical expertise and resources, posing a substantial challenge to scalability.
Infrastructure Limitations
AI solutions often demand high computational power and storage, which can strain existing resources.
3. Organizational and Cultural Challenges
Challenges:
Skills Gap
There is a shortage of skilled AI professionals, making it difficult to develop and implement AI solutions.
Resistance to Change
Employees may resist AI adoption due to fear of job displacement or unfamiliarity with new technologies.
Siloed Thinking
AI requires collaboration between departments, but organizational siloes can impede cross-functional cooperation.
4. Ethical and Societal Challenges
Challenges:
Bias and Fairness
AI models can perpetuate biases in data, leading to unfair or discriminatory outcomes. Especially, AI bias in helathcare can lead to devastating results and mistreatments.
Transparency & Accountability
AI systems, especially black-box models, can be difficult to interpret, making it challenging to ensure accountability.
Learn more about why Ethical AI in healthcare is a game-changer.
The Real Cost of Not Optimizing AI in Healthcare
5. Business and Strategic Challenges
Challenges:
Defining Clear Objectives
Without clear business goals, it’s difficult to measure the impact of AI or justify its investment. Also, lack of clear objectives further impose challenges on Artificial Intelligence strategy scalability.
Return on Investment (ROI):
Demonstrating the financial return on AI investments can be challenging, particularly in the early stages.
Check out how to calculate ROI of AI in healthcare industry.
AI scaling requires addressing these challenges with strategic planning, cross-department collaboration, and continuous investment in training, data management, and technological infrastructure. By overcoming these barriers, organizations can unlock the full potential of AI, driving innovation, efficiency, and sustainable growth.
How to Scale AI in Healthcare: A Framework for Gradual AI Scaling in Clinical Settings
AI scaling in healthcare, especially in clinical settings like radiology, is not an overnight process. It requires a strategic, gradual approach to ensure seamless integration, minimize disruption, and maximize the impact on patient care and operational efficiency. Here’s a structured framework for scaling AI in healthcare:
Step 1: Start Small with Pilot Projects
The journey to AI scalability in clinical settings should begin with pilot projects that test the technology’s capabilities and its potential to solve specific clinical problems.
Actionable Steps:
Choose a High-Impact Use Case
In radiology, this might involve testing AI for detecting lung cancer in CT scans or automated image analysis for fractures in X-rays.
Set Clear Goals and Metrics
Measure success based on diagnostic accuracy, time saved, and improvement in patient outcomes.
Involve Key Stakeholders
Engage radiologists and clinical staff in the pilot phase to gain their input and ensure the AI tool is aligned with real-world clinical workflows.
Why It Works:Â
Starting small allows organizations to evaluate AI’s effectiveness and ROI before scaling it across the entire department. It also allows for real-time feedback, which helps refine the technology and enhance its clinical value.
Step 2: Expand Gradually Across Departments
Once the pilot shows success, the next step is to scale AI across other clinical areas.
Actionable Steps:
Gradual Rollout
Move from one department or use case (e.g., radiology) to other diagnostic areas (e.g., cardiology, neurology). Ensure interoperability between AI tools and existing clinical systems (PACS, RIS, EHR).
Refine AI Models:
Use feedback loops from initial AI use to fine-tune models and expand their applicability across other clinical areas.
Provide Continuous Training
Ongoing staff training ensures staff confidence in using AI and maximizes the system's utility.
Why It Works:Â
Gradual expansion helps ensure that AI integrates smoothly into clinical workflows, reduces resistance from staff, and ensures that the technology continues to meet clinical needs effectively.
Step 3: Achieve Enterprise-Wide AI Integration
Finally, when the AI tools are successfully operating in multiple departments, enterprise-level integration can be pursued.
Actionable Steps:
Centralize Data Management
Ensure all AI tools work with a unified data system, integrating seamlessly with EHRs and imaging systems for comprehensive patient care.
Ensure Scalability
Use cloud-based platforms that can handle increasing data volumes as more departments are brought on board.
Monitor and Optimize
Continuously track the performance and efficiency of AI systems, optimizing them based on real-world clinical outcomes and operational metrics.
Why It Works:Â
Full-scale AI integration allows healthcare organizations to optimize operations, reduce clinical errors, and provide faster diagnoses. At this stage, AI becomes a critical tool for the healthcare system’s long-term success.
AI success depends on recognizing the 10-20-70 rule, which says that unlocking value means focusing 10% of effort and resources on building new algorithms, 20% on the underlying technology platforms, and 70% on investments in people, processes, and the transformation of the organization. [BCG Report]
Get access to our latest whitepaper to learn strategies on Maximizing AI impact on healthcare
70% of healthcare organizations are still in the early stage of AI implementation and struggling with use case identification and scaling.Â
Download this whitepaper to learn more about:
- How to identify use cases in healthcare
- Strategic approach to AI implementation
- How to maximize healthcare ROI
- Understanding the 70-20-10 principle
Future of AI Scaling Strategies: AI-as-a-Service Models
The rise of cloud computing has revolutionized the way healthcare organizations can scale their AI initiatives. Traditionally, scaling AI strategies required heavy investment in infrastructure—data centers, high-powered servers, and specialized hardware. However, cloud-based AI tools offer a more cost-effective and scalable solution.
What Is AI-as-a-Service?
AI-as-a-Service (AIaaS) refers to the cloud-based delivery of AI tools and services that allow healthcare organizations to access AI models without having to develop or host them internally. With AIaaS, organizations can leverage ready-made models and platforms for machine learning, data analytics, and natural language processing.
Why Cloud-Based AI Is Essential for Healthcare:
Scaling without Investment in Infrastructure
AI-as-a-Service models provide the ability to scale AIÂ strategies across departments without heavy infrastructure investments, allowing smaller healthcare providers to adopt AI technologies previously available only to large organizations.
Continuous Updates and Improvements
Cloud-based AI platforms are continuously updated and optimized by service providers, ensuring that healthcare organizations always have access to the latest AI innovations without having to manage these updates internally.
Conclusion: AI as a Strategic Investment for Radiology Departments
The scaling of AI in healthcare holds immense potential, but it requires a thoughtful and strategic approach. By starting small with pilot projects, leveraging cloud-based AI tools, and addressing ethical concerns, healthcare organizations can unlock the power of AI to improve patient outcomes, reduce costs, and enhance operational efficiency.
Adopting AI solutions with a focus on scalability, trust, and ethical responsibility ensures that healthcare providers can harness AI’s benefits while safeguarding patient trust and improving the quality of care. As AI continues to advance, scaling it effectively will be crucial for organizations looking to innovate, grow, and remain competitive in an ever-changing healthcare landscape.
Are you looking for a trusted AI strategy consulting company in the USA? Get in touch with Amzur Technologies now and build scalable AI strategies for your healthcare business.Â
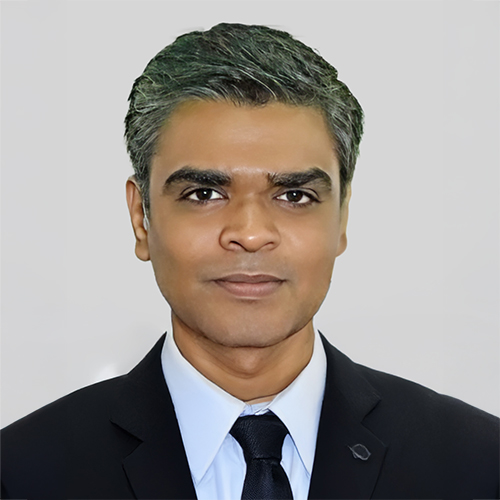
Director ATG & AI Practice