A Practical Guide To AI Implementation Strategy For SMBs
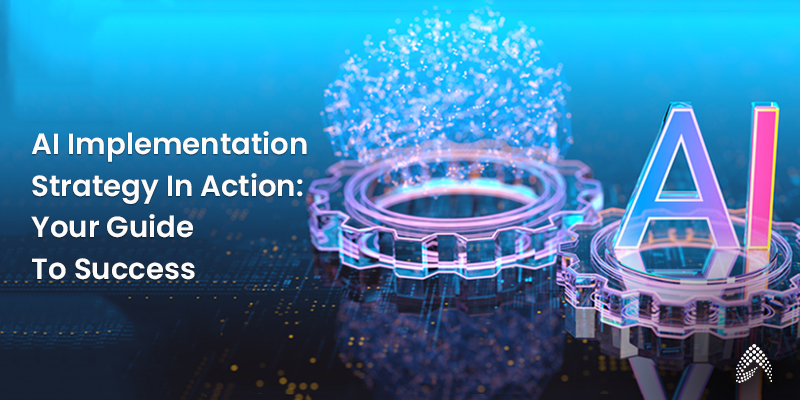
With the proliferation of data and technological maturity across industries, AI implementation has become a lynchpin in bringing new levels of automation, consistency, scalability, and reduced costs.
Regardless of the size and scale of businesses, AI implementation rates have skyrocketed in recent years, transforming the way organizations look at their business data and use it for a better customer experience and internal operational productivity.
According to many reports from giants like Gartner and McKinsey, over 50% of AI initiatives struggle to meet the organization’s objectives due to a lack of strategy and a clear roadmap.
In this article, you will find:
- Defining Clear Business Objectives
- Align AI strategies with defined business objectives
- Prioritize Objectives Based on Immediate Value and ROI
- Ensuring Data Readiness
- Selecting the right AI solutions for your organization
- Resource Planning & Training and Budgeting
- Conduct Proof-of-Concept Tests
- Monitoring KPIs and AI implementation success factors
- Governance and Compliance
C-level executive’s concerns about AI implementation:
Though AI implementation promises improved productivity, reduced time to market, and collaboration, many business leaders are still concerned about security & privacy with AI solutions.
Above all, scaling artificial intelligence implementation in the next 5 years has become a daunting challenge for many C-level executives.
C-level executives want to know if they should act now – and, if so, how to start. Some may see an opportunity to leapfrog the competition by revamping their processes and delivering innovative applications and value faster. Others may want to exercise and experiment with a few use cases and learn more before making larger investments.
This blog will help you learn how to build a custom AI implementation strategy to meet your business objectives.
AI Implementation For Small Businesses:
For successful AI implementation, organizations must focus more on business challenges and how AI can add value to their processes and meet objectives.
1. Defining Clear Business Objectives:
The AI implementation journey starts with a clear vision and an end goal. Clear articulation of the organization’s goals and how AI aligns with them would significantly save time, cost, and resources.
Whether optimizing processes, accelerating development time, enhancing customer experience, or multiplying your team’s productivity will set the trajectory for successful AI adoption.
Beginning with the end in mind can maximize your AI implementation potential and ensure better ROI.
Consider you are relying on employees to manually administer claims or invoice processing, which is a tedious and error-prone activity. Here, your biggest challenges are tedious task automation and improving your employee’s productivity.
2. Align AI strategies with defined business objectives:
Once potential AI initiatives have been identified, the next step is to prioritize them based on their strategic business goals and potential Return on Investment (ROI).
This involves identifying opportunities where AI can:
1. Automate Repetitive Tasks:
AI-based automation streamlines repetitive tasks, such as data entry, document verification, and claim processing, allowing your team to focus more on delivering a better customer experience. NLP techniques enable AI to extract relevant information from unstructured data, expediting the assessment process and minimizing human error. This automation optimizes resource allocation and accelerates the overall process.
2. Enhance Decision-Making:
AI tools are trained to classify and organize structured and unstructured data to predict future outcomes based on existing patterns. This can help your company:
- Make more data-informed decisions
- Identify new business opportunities
- Better understanding of your customers and personalizing your marketing approach
- Prepare for potential challenges.
You could use this data to improve your product launches, customer experience, marketing, and hiring strategies.
3. Research and development:
AI is a great enabler to continue innovation and stay ahead of the competition. AI helps in understanding nearly any industry by collecting and analyzing tremendous amounts of information efficiently and accurately. This can help businesses research and develop problem-specific solutions.
Incorporating AI strategies and solutions into your R&D provides a competitive edge by eliminating tedious tasks, accelerating time-to-market, fostering innovation, and staying ahead in the dynamic market.
4. IT Operations:
AI’s role in IT operations is inevitable and helps in analyzing system log file errors and identifying issues before they become fatal. Gartner defines AIOps as “the application of machine learning and data science to IT operations problems.” It can identify issues so the IT team can proactively safeguard and fix them before any IT system goes down and disrupts operations.
5. HR Operations:
Think about your HR team – which involves a lot of manual and tedious work day in and out. It’s checking emails, reaching out to new candidates, scheduling interviews, following up, and onboarding them.
Consider how much time your team spends on these tasks – from planning to screening and onboarding. It takes up a lot of your work day. Artificial Intelligence solutions adoption can help your HR team in many ways:
- Candidate sourcing from various job boards and resume screening
- Sending automated reminders to interviewers and candidates based on their availability
- Automating paperwork, providing digital assistance, and ensuring a smooth onboarding process
- AI helps in personalizing training programs, suggests courses, and helps in enhancing employee skills
- AI-driven analytics process HR data and help in strategic decision-making
A&F operations involve a lot of human intervention and tedious tasks. In fact, it’s one of the tedious jobs for many experts with old-fashioned methodologies. On the other hand, AI strategies for accounting and finance operations can save you a lot of time, resources, and cost.
AI helps in workflow management – reducing tedious data entry and invoice processing operations and human errors. Further, AI can assist in ensuring compliance with regulatory standards by automating compliance checks and audits, reducing the risk of non-compliance and associated penalties.
7. Customer Services Operations:
AI technology is the enabler and gateway toward digital transformation for companies providing continuous customer services.
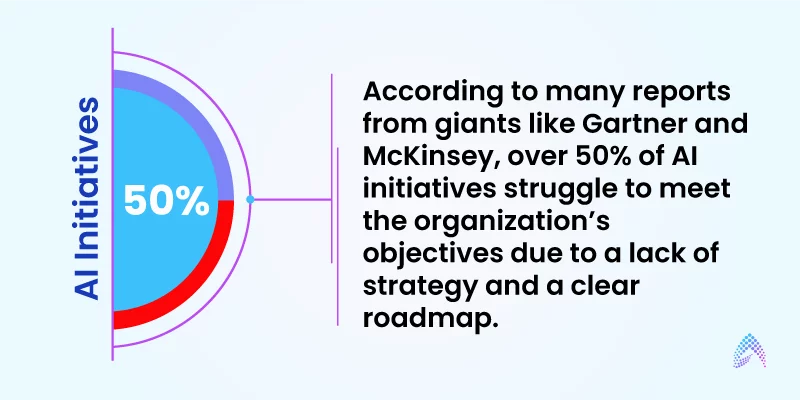
Leveraging AI tools to develop NLP bots and integrating them with APIs can enhance real-time customer service and save costs.
8. Personalization:
These days, personalization wins over any other marketing strategy. YouTube and Spotify will recommend based on your history. Amazon will remind you that it’s time to buy your monthly groceries and other products. If you are planning to personalize your marketing and sales approach, AI navigate you through the journey.
AI personalization utilizes data, customer engagement, deep learning, natural language processing, machine learning, and more to curate highly tailored experiences for end-users and customers.
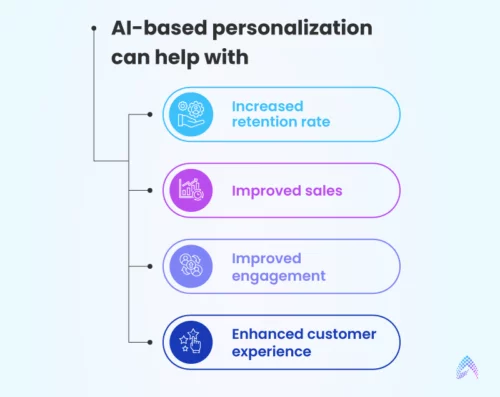
9. Quality Assurance:
Organizations are moving from manual to automated to AI-powered testing methodologies to ensure better quality and performance. AI-enabled testing tools can automate test case generation, execution, and analysis, accelerating product testing and ensuring robust quality checks.
This further helps in identifying malicious patterns, anomaly detection, and root cause analysis to resolve issues for continuous improvement.
AI strategies for QA ensure consistent monitoring and assessment, allowing you to develop high-precision products.
3. Prioritize Objectives Based on Immediate Value and ROI:
Some high-value delivering processes need immediate automation, and others can be automated later. In your Artificial Intelligence implementation strategy, prioritize projects based on their alignment with strategic objectives, potential impact, and estimated ROI.
Assess the technical feasibility, resource requirements, and potential impact on overall performance and productivity. Consider factors such as data availability, integration with existing systems, and the likelihood of successful implementation.
These objectives could be something like:
2. % of improvement in customer satisfaction/ engagement
3. Error reduction and reworking rate in your SDLC
Example:
Enhanced customer experience is the need of the hour. Henceforth, you can incorporate AI-powered chatbots to immediately address customer support/ engagement challenges and expect a better ROI regarding nurturing and long-term relationships.
AI chatbots not only improve customer experience but also optimize resources and productivity. Some automation tools can enable businesses to integrate with other applications like customer relationship management (CRM) software, creating a more efficient and streamlined workflow.
4. Ensuring Data Readiness:
Data is the lifeblood of all businesses across industries. But what if you are operating in silos with low-quality data?
On the other hand, only 4% of respondents said their data is fully accessible to make informed decisions.
The success of AI strategy adoption hinges on the quality of data. AI implementation helps in auditing existing data sources to clean data, remove duplicates, resolve missing values, and lay a robust foundation for accurate AI-driven analysis.
Leveraging advanced analytical tools unlocks valuable insights from complex datasets, empowering organizations to make informed decisions.
Here are some areas where you need high-quality data to make informed decisions.
- Fraud Detection: Here, high-quality data ensures accurate patterns for AI algorithms to detect anomalies, enhancing fraud detection accuracy.
- Customer Analytics: In the digital age, having the right customer data and customer behavior patterns allows you to drive personalized strategies and foster improved customer relationships.
- Predictive Maintenance: High-quality data is vital in enabling precise predictive models. AI further helps in optimizing maintenance schedules and reducing downtimes.
- Change Management: Training AI/ML tools with high-quality data aids in assessing organizational needs and change impacts, and provides insights for smoother change implementation and adaptation.
- Compliance: If you’re operating in different regions, high-quality data is crucial for accurate compliance checks, ensuring adherence to stringent country-specific regulations.
- Data Integration and Modeling: Good data is the backbone of accurate business operations. Training AI tools with good data delivers precise insights to create new business models and make informed decisions.
- Supply Chain Management: Training AI tools with high-quality data helps in optimizing supply chain operations by forecasting demand, improving logistics, minimizing inventory costs, and enhancing overall supply chain efficiency.
- Preventive Maintenance and Support: Providing historical data to the AI tools can empower you to identify potential issues, enabling proactive maintenance and support, reducing downtime, and enhancing reliability.
Example:
Managing extensive data sets and diverse formats and ensuring adherence to privacy regulations presents a significant challenge for many businesses.
Implementing AI-powered Language Models (LLMs) such as ChatGPT, Bard, and others can streamline data summaries, expediting decision-making processes across various domains. Additionally, integrating backend engines with Cloud platforms via APIs facilitates smoother data migration and integration.
This approach minimizes processing delays and enhances decision-making speed, fostering a data-driven transformation for businesses across industries.
5. Selecting the right AI solutions for your organization:
AI implementation is a game-changer in automating business operations and delivering value to customers. However, with a plethora of AI tools available, selecting the right one for your specific needs can be a daunting task.
From Machine Learning to Natural Language Processing tools and Robotic Process Automation, you need a strategic approach to finding the optimal fit that aligns with your business needs. While selecting your AI solution, remember the One-size-fits-all approach won’t work.
Let’s look at the use cases of each AI solution.
1. NLP:
It facilitates the creation of intelligent chatbots capable of understanding, analyzing, and responding to natural language. These bots can handle customer inquiries, provide instant support, and guide users through processes. This further helps in enhancing customer service efficiency 24*7 and reduces manual intervention
2. Machine Learning:
ML algorithms and models are being used to continuously learn from historical and new data, enabling organizations to identify and mitigate fraudulent operations in real-time.
In the insurance industry, ML algorithms analyze various data points within incoming insurance claims, including claimant information, policy history, claim details, and associated documents. If a claim exhibits unusual characteristics such as inconsistent information or exaggerated damages, the ML model flags it as potentially fraudulent, saving millions of dollars.
3. Predictive Analytics:
AI-powered predictive analytics offer significant benefits to optimize processes, improve efficiency, and enhance decision-making capabilities.
Imagine you are in the energy industry and managing a utility company responsible for maintaining a network of power grids.
For you, AI-powered predictive analytics helps gather vast amounts of data from sensors embedded in power grid infrastructure and information on equipment health, temperature, voltage, current flow, and more.
Together with ML algorithms, you can utilize this data to identify patterns and trends that indicate potential equipment failures or unprecedented downtimes. For instance, you can replace a transformer based on the predicted failure probability, thus avoiding costly unplanned downtime.
4. Robotic Process Automation (RPA):
If speed and accuracy are your top priorities, then RPA helps you significantly reduce the time taken to automate tedious tasks. RPA implementation minimizes human intervention and errors that occur in manual data entry.
RPA bots extract information from the claim form, email, or uploaded documents when a new claim is submitted. If you have a CRM like NetSuite, data can be automatically uploaded into the company’s system, eliminating manual data entry.
Then, RPA bots validate the received information against predefined rules and databases. For instance, they can cross-check claimant details against the company’s policy database to verify coverage details.
5. Computer Vision:
AI-powered computer vision algorithms identify irregular patterns or anomalies in visual data, particularly in manufacturing or quality control operations. This technology ensures better precision in anomaly identification, fault prevention, and ensuring minimal defects. Analyze past data and patterns to predict equipment failure or security breaches, allowing for preventative maintenance and risk mitigation.
6. Deep Learning:
Deep Learning models, such as Recurrent Neural Networks or Transformer-based architectures like BERT, can process textual information within documents.
These models can understand the context, structure, and semantics of text, facilitating text extractions, entity recognition, and even summarizing information from documents like invoices, forms, or reports, streamlining workflows and saving valuable time.
7. GenAI:
GenAI will truly revolutionize product development by analyzing data and customer behavior to forecast future demands and develop products that resonate with the market. This allows you to adapt quickly to market demands and deliver future-ready solutions.
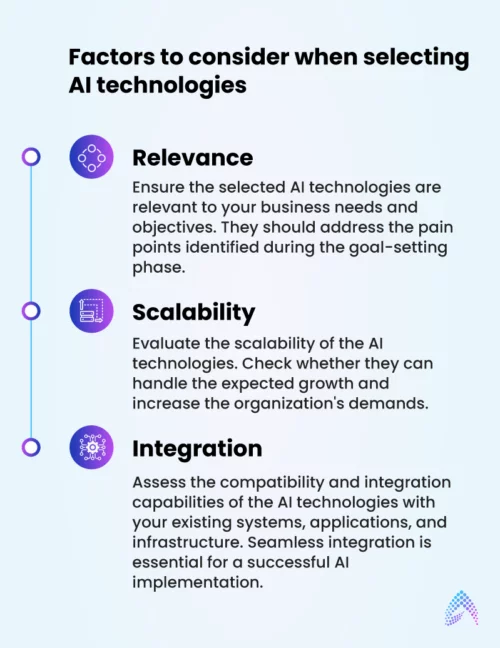
6. Resource Planning & Training and Budgeting:
Along with defined objectives, technologies, and tools, having the right team is essential to win the AI implementation game.
But AI strategy implementation will raise two concerns for organizations:
- Where do we get qualified AI experts?
- How do we train and make them acquainted with the ongoing changes?
With talent being one of the biggest challenges to AI adoption, most organizations say they lack the in-house expertise to create, deploy, and manage AI projects at scale.
Organizations are relying on hiring external talent, building in-house capabilities, and buying or licensing capabilities from large technology firms to meet their AI implementation goals.
Even the early adopters or tech giants report a mix of internal and external sourcing. They are hiring external resources and building in-house teams by investing in upskilling and incorporating modern skills.
On the other hand, APIs can help you accelerate the deployment and integration of AI-powered applications with existing systems, eliminating the need to build from scratch.
Identifying the right team and building a sound foundation to explore AI can help you tap new business opportunities while expediting your team’s productivity.
To collaborate with a third-party AI services provider, vet their experience and industry-specific expertise, and onboard them.
Budgeting:
AI project implementation budget involves estimating the costs associated with acquiring AI technologies, hiring talent, training employees, infrastructure setup, software licenses, ongoing maintenance, and potential scalability. A well-defined budget eliminates financial losses and ensures project viability.
7. Conduct Proof-of-Concept Tests:
Based on your priorities and business needs, start pilot projects to gauge the performance and suitability of AI solutions. Then, with insights into how each solution aligns with your specific requirements, you can scale projects further.
Start small and scale your AI implementation. Fine-tune algorithms, address performance issues and incorporate user feedback into your solution.
According to an Accenture report, Achievers devoted 14% of their total technology budgets to AI, while in 2021 they devoted 28%. In 2024, they plan to devote 34%.
To avoid being left behind, companies are investing small and increasing their spending in the long run.
By thoroughly testing and piloting your AI implementation, you can ensure that it meets the desired performance standards, addresses user needs, and delivers the expected outcomes.
8. Monitoring KPIs and AI implementation success factors:
In assessing AI systems effectiveness, establishing at least one KPI is crucial. For instance, improved conversion rates are KPIs for sales teams. Also, metrics like active user sessions, click frequencies, and overall interaction rates serve as valuable KPIs, reflecting user engagement.
Conduct user feedback through surveys, reviews, or direct channels. A high satisfaction score reflects the AI’s alignment with user needs. Monitoring such insights further helps you adjust AI strategies and optimize organizational value.
Allocate dedicated time every month or quarter to gauge the AI tool’s performance against set goals:
- Track the tool’s usage for its intended purpose and identify any emerging patterns in its application.
- Encourage teams to innovate and find new ways to leverage the tool’s capabilities.
- Assess the quality of the tool’s outputs—identify areas where manual intervention or improvements might be necessary.
- Solicit feedback from customers or vendors interacting with AI-generated outputs to gauge sentiment and make necessary adjustments.
- Measure the time saved by employing AI within workflows and compare it against initial expectations.
Later, focus on continuous improvement. You can revise, refine, change, and set new KPIs for your teams, ensuring scalability and a better ROI.
9. Governance and Compliance:
Governance and compliance frameworks are essential pillars safeguarding against bias, ensuring legal compliance, mitigating risks, and building trust. They promote ethical outcomes, legal adherence, and transparent decision-making. They also establish accountability and transparency, enabling stakeholders to comprehend and scrutinize AI-driven decisions, and fostering trust among users and stakeholders by assuring the quality, reliability, and ethical integrity of AI systems.
Amzur’s AI implementation framework:
Amzur’s comprehensive and result-driven AI framework consists 5 stages:
1. Assessing AI maturity for competitive strategy:
We assess and understand whether your AI capabilities stand with the industry benchmarks. Also, we do a SWOT analysis and make informed decisions about AI investment priorities. We measure the degree to which organizations have mastered AI-related capabilities in the right combination to achieve high performance for customers, shareholders, and employees.
2. Aligning AI deployment with business objectives:
We ensure that AI initiatives directly support and complement overarching business goals, enhancing efficiency and contributing to delivering meaningful outcomes.
3. Seamless AI-integration strategies:
We address data silos and ensure data is ready for the AI model and integrate it seamlessly into existing workflows and systems while minimizing disruptions.
4. Cost-effective AI solutions to maximize ROI:
In our AI implementation strategy, we value our client’s budget and identify the best Cloud-based AI tools to reduce initial costs. We ensure to maintain budget discipline through continuous ROI assessment.
Ex: Microsoft Azure Machine Learning: This robust platform provides a comprehensive set of tools and services for building, deploying, and managing AI models, enabling tailored solutions for specific cloud needs.
5. The roadmap to AI-powered transformation:
We develop a clear AI strategy that aligns with your business goals and build a strong AI team with diverse expertise. Investing in data infrastructure and governance enables a culture of continuous learning and improvement.
Case Study:
In today’s healthcare landscape, data overload, and manual processes can hinder efficient diagnosis and optimal patient care. Our client needed help with a high volume of patient reports, time-consuming manual reviews, and stringent data privacy regulations.
Amzur partnered with the organization to implement a customized AI strategy to revolutionize its data processing and decision-making capabilities. Our laser-focused approach to workflow automation and data silo elimination enabled significant gains, paving the way for long-term scalability and a more patient-centric healthcare experience.
Watch our on-demand webinar to discover how Amzur’s AI strategy empowered this healthcare organization to:
- Substantially reduce patient report processing time
- Eliminate time-consuming manual review processes
- Ensure seamless data accessibility and compliance
- Scale operations efficiently for future growth
Conclusion:
By investing in robust AI implementation strategies for SMBs, you can ensure that your organization is well-positioned and equipped to navigate the ever-changing landscape of technology and remain competitive in the long run. Start assessing and building robust and scalable AI business strategies to unlock potential innovation and growth.
FAQs
There’s no “one-size-fits-all” solution. Evaluate your specific needs and challenges, consider available resources, and research existing AI tools and technologies. Consult with experts if needed to find the best fit for your organization.
Address talent gaps by upskilling existing employees, leveraging partnerships with AI consultancies, or seeking freelance expertise. Optimize costs by exploring open-source tools, cloud-based solutions, and scalable infrastructure options.
Establish clear ethical guidelines, prioritize data privacy and security, and implement human oversight mechanisms. Monitor for potential biases in algorithms and strive for transparency in AI decision-making processes.
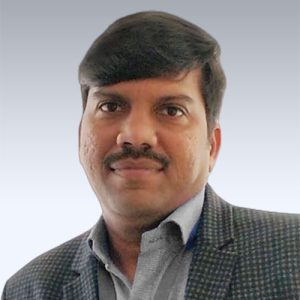
Director – Global Delivery