AI in Healthcare Industry: How to Tackle AI Implementation Issues
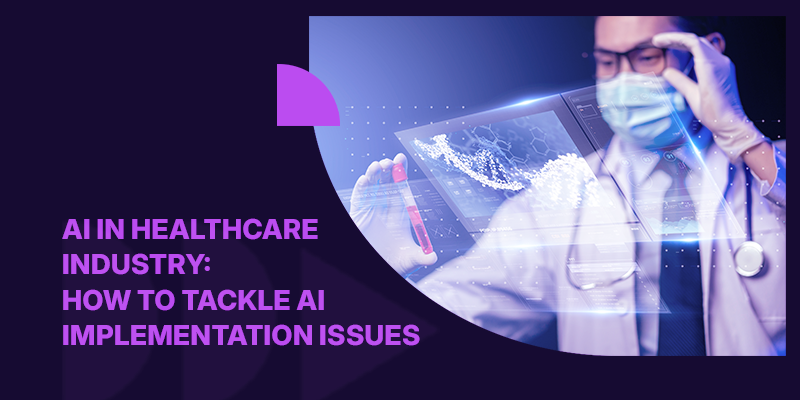
In 2024, a clear shift emerged in healthcare’s relationship with AI. A staggering 42% of all global digital health funding flowed into AI-focused companies—up from just 7% in 2015—signaling a tipping point in both investor confidence and industry interest. AI in healthcare industry has transformed how doctors interact with patients and provided personalized care without disruptions. [Statista – AI share in healthcare]
Yet, despite the capital and curiosity, most U.S. healthcare organizations remain early in their AI journey. Roughly one in five are still experimenting, with no production use cases. While 11% report mid-stage adoption with multiple AI models in production, and 14% are in early stages, the real momentum is seen in generative AI, where 46% have begun initial production implementation—a sign of pragmatic acceleration.
This blog discusses the challenges of AI implementation in healthcare and its solutions
AI Implementation in Healthcare Industry Concerns Among Clinicians and Patients:
Clinicians reflect a nuanced reality. While over a quarter of those familiar with AI have used it for work, widespread usage remains cautious. Globally, there’s measured optimism about AI’s promise, but also deep-rooted concerns. Four in ten doctors and one in four nurses see AI in healthcare industry as a helpful evolution of their roles. However, ethical concerns dominate, especially in Europe, where nearly 60% of clinicians report serious reservations.
Patients, too, are wary. The most cited fear? That AI might erode the human connection in care—closely followed by data privacy risks.
What this tells us: The future of AI in healthcare industry is not just about algorithms—it’s about trust, transparency, and targeted implementation. Organizations must move beyond experimentation, address ethical AI and relational concerns, and design AI that enhances human care.
This article explores major implementation challenges of AI in healthcare industry and a framework for achieving successful AI integration. Whether you’re a CIO, health system leader, or IT strategist, this guide will help you navigate the nuanced path from AI ambition to reality.
AI Implementation In Healthcare: Challenges and Solutions
The case of AI in radiology and image analysis is an excellent example of how artificial intelligence in healthcare can improve and streamline patient care, diagnostic procedures, and more. However, it is also evident that AI in healthcare industry comes with a host of challenges.
Let’s look at the top AI adoption challenges in healthcare and solutions.
1. Bias in AI Algorithms:
AI models can be biased due to the data they are trained on, leading to a skewed AI’s promise hinges on fairness, but biased algorithms threaten to undermine it. When models are trained on skewed datasets, they can perpetuate racial, gender, or socioeconomic disparities, amplifying existing inequities in healthcare.
Imagine a diagnostic tool that misjudges conditions in minority groups or an AI program blind to social determinants of health—the consequences are dire: unequal access, poorer outcomes, and entrenched systemic injustice.
The Solution:
Combat bias head-on by prioritizing diverse, representative datasets and rigorous testing. Healthcare leaders must invest in continuous auditing of AI outputs, partnering with ethicists and data scientists to root out disparities. Transparency in algorithm design isn’t just a safeguard—it’s a moral imperative that builds trust and delivers equitable care.
2. Data quality and accessibility:
AI thrives on high-quality, standardized data, yet healthcare’s reality is far messier. Siloed records, inconsistent formats, and interoperability gaps between Electronic Health Records (EHRs) hobble AI’s accuracy and credibility. With healthcare data projected to grow at a staggering 36% CAGR by 2025, the opportunity is immense—but so is the challenge. Poor data quality isn’t just a technical hiccup; it’s a barrier to life-saving insights. [Source]
The Solution:
Break down silos with robust data governance and interoperability standards. Invest in data cleansing and integration platforms to transform fragmented records into a unified, AI-ready resource. Organizations like the Cleveland Clinic prove it’s possible—by leveraging AI to optimize patient flow, they’ve slashed wait times by 10%. Quality data isn’t a luxury; it’s the foundation of AI success.
3. Data Security and Privacy Concerns:
AI’s ability to uncover hidden health patterns is awe-inspiring—60% of healthcare leaders in the 2024 Medscape & HIMSS report laud its diagnostic prowess. Yet, 72% flag data privacy as a towering risk. In an era of escalating cyber threats, protecting sensitive patient information isn’t optional—it’s non-negotiable.
The Solution:
Fortify defenses with cutting-edge encryption, strict access controls, and compliance with regulations like HIPAA. Proactive risk assessments and staff training can preempt breaches, while transparent privacy policies reassure patients and providers alike. Security isn’t a cost—it’s the bedrock of AI-driven trust. [Source]
4. Cost and Acceptability Constraints
AI in healthcare industry demands hefty upfront investments—upgraded infrastructure, training, and compliance don’t come cheap. For smaller clinics, the price tag can feel insurmountable. Meanwhile, skepticism lingers: a 2023 study reveals clinical staff resist AI, wary of new skills and complexity, slowing its adoption. [Source]
The Solution:
Start smart with scalable, cost-effective solutions—think cloud-based AI tools or partnerships with experienced vendors to sidestep wasteful missteps. Cleveland Clinic’s success shows that strategic investments pay off. For acceptability, prioritize user-friendly interfaces and hands-on training to empower staff, turning reluctance into enthusiasm. The payoff? Faster diagnoses and better outcomes.
Real-time Example
Cleveland Clinic has been at the forefront of implementing AI in healthcare industry and overcoming data quality issues. By successfully implementing AI solutions to analyze patient flow and optimize scheduling, they’ve reduced wait times by 10%.
Yet, achieving this level of AI implementation in healthcare requires significant investment in technological infrastructure, regulatory compliance, staff training, and more. Organizations can mitigate these financial barriers by using trusted, experienced resources that reduce the cost of implementation and avoid wasteful steps.
5. Integration with Legacy Systems
Legacy healthcare systems and outdated infrastructure can hinder the seamless integration of AI technologies into existing workflows, slowing adoption and effectiveness. The slow adoption of AI in healthcare industry could limit its potential benefits, causing delays in the application of AI-driven interventions and underutilization of valuable AI-generated insights.
The Solution:
Embrace phased modernization. Bridge gaps with middleware that connects old and new, allowing AI solutions to enhance workflows without a full overhaul. Forward-thinking organizations treat AI implementation in healthcare as an investment in agility, not a burden, unlocking AI’s insights without disruption.
6. Skills Gap and Training Needs
AI in healthcare industry demands expertise—data scientists, clinical informaticists, and strategic leaders like the emerging Chief AI Officer (CAIO). Yet, a 2023 GlobalData study highlights a glaring skills shortage. Without the right talent, AI initiatives falter.
The Solution:
Close the gap with targeted training programs and partnerships with AI experts. Upskill existing staff and recruit specialists to build in-house mastery. A skilled workforce doesn’t just implement AI—it maximizes its business and clinical value, confidently driving innovation. [Source]
7. Ethical, Legal, and Regulatory Uncertainty
Questions about data privacy, algorithmic bias, and accountability remain largely unanswered. Regulations like HIPAA in the U.S. provide a baseline, but the legal framework around AI and healthcare is still evolving, creating uncertainty for healthcare leaders.
Often, implementation of AI in healthcare industry lack clear lines of accountability, especially in critical life-and-death decision-making scenarios. There’s often uncertainty about who is responsible for AI outcomes. Failure to ensure accountability in AI applications could lead to medical errors, misdiagnoses, and challenges in regulating ethical AI in critical health decisions.
The Solution:
Stay ahead with proactive compliance frameworks and clear accountability protocols. Engage legal and ethical experts to shape policies that balance innovation with responsibility. Clarity here isn’t just regulatory—it’s a competitive edge that fosters trust and adoption.
AI Solutions In Healthcare: How To Make It Work
Ready to bring AI into your healthcare ecosystem? This isn’t just a plan—it’s a launchpad for innovation, blending practicality with vision. Let’s dive into the steps that will turn AI implementation in healthcare into a game-changer.
Step 1: Set Your Goals First—Define Success with Precision
Implementing AI in healthcare industry isn’t about tech for tech’s sake; it’s about results. Start by pinpointing what “winning” looks like—faster diagnoses? Reduced readmissions? Cost savings? Tie every metric to patient outcomes (e.g., 20% faster MRI analysis) and organizational goals (e.g., 15% lower operational costs).
Involve executives, clinicians, and even patients to ensure your KPIs resonate across the board. This isn’t just a step; it’s your foundation.
Step 2: Master Your Data—Clean, Curate, Conquer
AI is only as good as the data it feeds on. Dive into your datasets—EHRs, imaging archives, billing records—and scrub them clean. Fix inconsistencies, fill gaps, and standardize formats. Don’t have enough data? Partner with an external AI solutions provider or synthetic data providers.
Step 3: Rally the Team—Engage Clinicians and Stakeholders Early
AI in healthcare industry doesn’t work in a vacuum—it needs champions. Bring clinicians, nurses, and IT staff into the fold from day one. Their insights will shape practical solutions (e.g., “This tool needs to fit our triage workflow”), while their buy-in will ease adoption.
Don’t stop there—loop in patients, too. A patient advisory panel can flag what matters most, like clear communication of AI-driven decisions. This isn’t just collaboration; it’s co-creation.
Step 4: Pick the Right Tools—Opt for Explainable, Auditable AI
Black-box AI won’t cut it in healthcare. Choose models that show their work—think decision trees or interpretable neural networks—so clinicians can trust and verify outputs. Auditability is non-negotiable; regulators and ethicists will demand it.
Test for bias (e.g., does it perform equally across demographics?) and document everything. This isn’t just about compliance—it’s about building a tool that stands up to scrutiny and saves lives.
Step 5: Keep Score—Monitor with Outcome-Driven KPIs
Launch day isn’t the finish line—it’s the starting gun. Track your success metrics relentlessly: Are patients recovering faster? Are costs dropping? Use dashboards for real-time insights and tweak as needed. Don’t just measure tech performance—gauge user satisfaction and patient trust, too. This isn’t maintenance; it’s evolution, ensuring AI delivers value every day.
Step 7: Partner Up—Leverage Expertise and Resources
You don’t need to reinvent the wheel. Team up with AI vendors or AI consulting service providers who’ve cracked the code. They’ll bring proven tools, cut costs, and dodge pitfalls. This isn’t outsourcing; it’s amplifying your impact.
Step 8: Plan for Scale—Build with Growth in Mind
Start small—a pilot in radiology, say—but design for the big leagues. Ensure your AI integrates with legacy systems (middleware can help) and scales across departments. Test cloud solutions for flexibility. This isn’t just foresight; it’s future-proofing your investment.
Step 9: Mind the Budget—Maximize ROI Without Breaking the Bank
Implementing AI in healthcare isn’t cheap, but it doesn’t have to bankrupt you. Start with high-impact, low-cost pilots (e.g., automating appointment scheduling, medical imaging analysis). Seek grants or shared-risk vendor deals. Measure ROI early—cost savings, efficiency gains—to justify scaling up. This isn’t penny-pinching; it’s strategic spending.
Check out how the University of California is trying to address the AI bias in healthcare diagnostics and predictive analytics with its new framework, BE-FAIR.
Cost vs. Value: Is the Implementation of AI in Healthcare Industry Worth It?
While the promise of AI in healthcare industry is undeniable, decision-makers must weigh its costs against measurable outcomes. Upfront investments can be substantial, covering infrastructure upgrades, data preparation, vendor selection, and compliance measures.
On the other hand, AI implementation in healthcare brings numerous benefits, including:
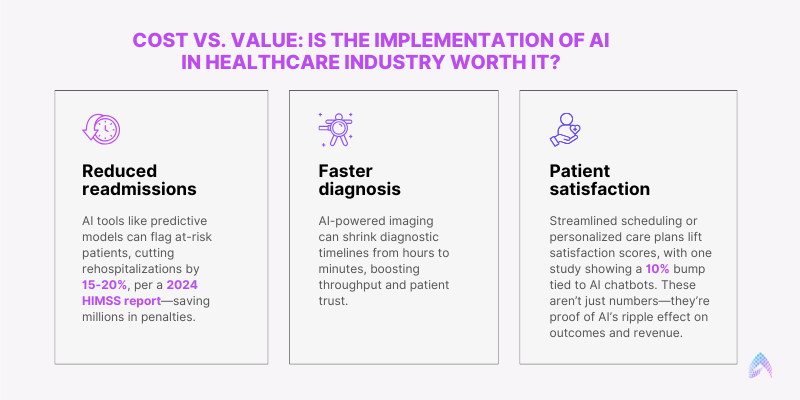
Conclusion: Why Partnering with the Right AI Solutions Provider Matters
Tackling the many facets of AI in healthcare industry requires more than technology—it takes vision, strategy, and trusted expertise. At Amzur, we provide tailored AI strategy consulting services that help healthcare organizations overcome integration barriers, ensure compliance, and achieve measurable outcomes. From strategy to execution, Amzur is your partner in unlocking the full potential of AI implementation in healthcare.
Maximizing AI's Impact In Healthcare: Implementation Strategies For ROI
70% of healthcare organizations are still in the early stage of AI implementation and struggling with use case identification and scaling.
Download this whitepaper to learn more about:
- How to identify use cases in healthcare
- Strategic approach to AI implementation
- How to maximize healthcare ROI
- Understanding the 70-20-10 principle
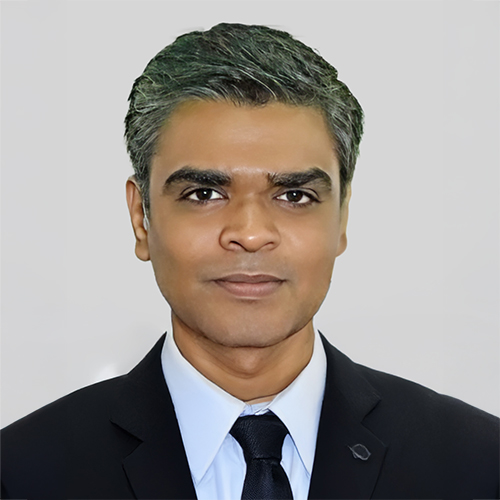
Director ATG & AI Practice