What AI Implementation Really Costs: A Comprehensive Guide
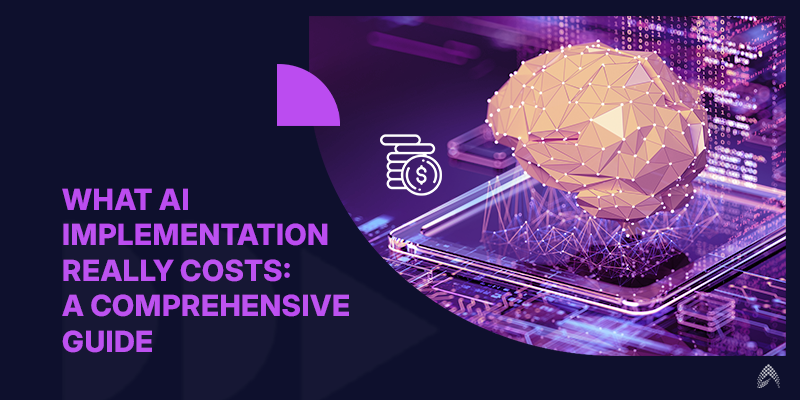
Artificial intelligence (AI) is transforming industries at an unprecedented pace, with global spending expected to reach $200 billion by 2025 (IDC). While 67% of executives believe AI will be vital for their business in the next two years (Deloitte), only 20% of companies have successfully scaled AI across multiple business units (McKinsey). This disparity between perceived importance and successful scaling highlights a critical issue: the true cost of AI implementation is often underestimated.
This blog delves beyond surface-level discussions to uncover hidden costs, overlooked benefits, and essential risk mitigation expenses tied to AI implementation. By gaining a comprehensive understanding of these factors, businesses can make informed decisions about their AI investments. From hidden expenses like data maintenance and employee training to unexpected advantages such as improved customer experiences and new revenue streams, AI implementation is a multifaceted process requiring careful cost-benefit analysis and a commitment to ethical deployment.
Total Cost Analysis: Beyond the Initial Investment
The true cost of AI implementation goes far beyond initial software and hardware expenses. Businesses must consider both direct (visible) and hidden (indirect/hidden) costs to understand the full financial commitment.
A. Direct Costs
These are the most visible and typically planned for in budgets:.Software and Hardware
Costs include AI platforms, GPUs for deep learning, and software licenses. Cloud-based solutions offer scalability but may result in higher long-term costs.
Example: Training large language models can require either expensive GPU servers or ongoing cloud-based fees.
Data Acquisition and Preparation
Preparing data for AI, including cleaning, labeling, and formatting, can be resource-intensive.
Example: A healthcare company annotating medical images for an AI diagnostic tool incurs significant costs.
Development and Integration
Building models, integrating AI with existing systems, and creating APIs involve salaries for AI engineers and developers.
Example: A retailer connecting an AI recommendation engine to its e-commerce platform incurs notable development expenses.
Consulting and Training
External expertise and employee training are often necessary for effective AI deployment.
Example: A manufacturer adopting predictive maintenance may hire consultants to assess infrastructure and train staff.
B. Hidden Costs
Often overlooked, these can significantly affect the total cost of ownership:Data Storage and Maintenance
Storing and managing vast datasets for AI requires significant infrastructure and security investments.
Example: A financial institution using AI for fraud detection faces substantial storage and maintenance costs.
Model Maintenance and Retraining
AI models need periodic updates to stay accurate and relevant, requiring ongoing expertise and resources.
Example: Chatbots must be retrained with new interactions to adapt to evolving customer needs.
Integration with Legacy Systems
Merging AI with outdated systems can require extensive custom development and upgrades.
Example: An enterprise updating its ERP system for AI-driven automation incurs significant IT expenses.
Change Management and Resistance
AI adoption can cause employee resistance, requiring investments in communication, training, and support.
Example: A logistics company introducing AI route optimization must address driver concerns and provide training.
Opportunity Costs
Implementing AI can divert resources from other strategic initiatives, potentially impacting revenue from delayed product launches or marketing campaigns.
Example: A marketing team focused on AI adoption may postpone other initiatives, potentially affecting revenue.
Unexpected Benefits: Beyond Efficiency Gains
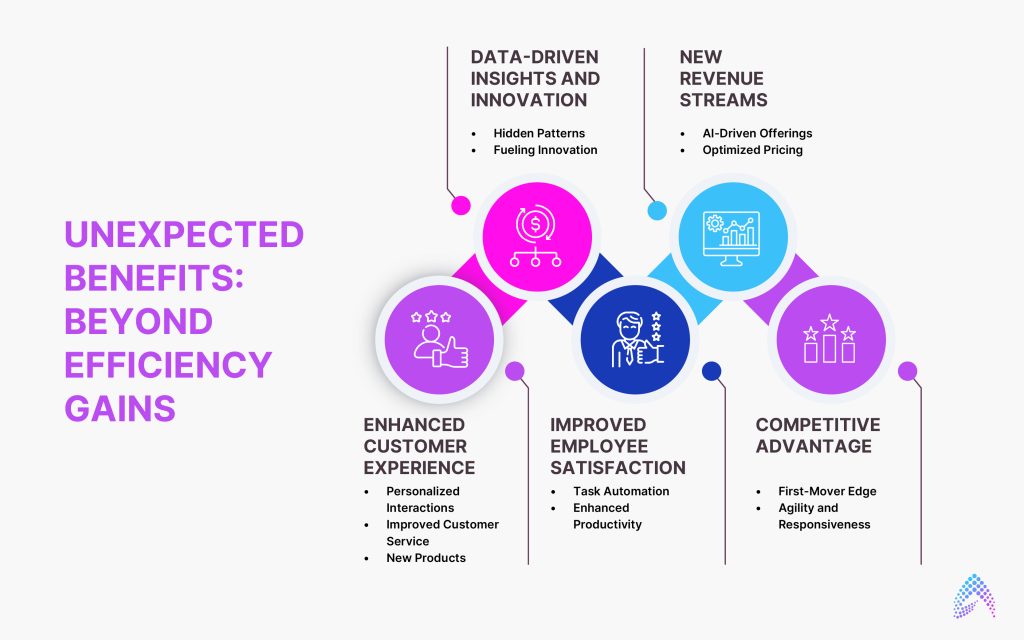
While AI adoption is often driven by cost savings and efficiency, it also unlocks unexpected advantages that elevate business value across various domains.
A. Enhanced Customer Experience
Personalized Interactions
AI analyzes customer data to deliver tailored recommendations and support, boosting engagement and loyalty.
Example: E-commerce platforms use AI-powered recommendations to increase conversions.
Improved Customer Service
AI chatbots provide 24/7 support, handling routine queries and freeing agents for complex issues.
Example: Banks use chatbots to reduce wait times and improve satisfaction.
New Products
AI enables innovative offerings, creating new revenue streams.
Example: Streaming services leverage AI for personalized content recommendations, enhancing user retention.
B. Data-Driven Insights and Innovation
Hidden Patterns
AI analyzes large datasets to uncover actionable insights, improving decision-making and strategies.
Example: Retailers optimize inventory and marketing using AI analysis.
Fueling Innovation
AI insights drive the creation of new products, services, and models.
Example: Pharma companies accelerate drug discovery with AI-based data analysis.
C. Improved Employee Satisfaction
Task Automation
AI automates mundane tasks, allowing employees to focus on strategic and creative work, reducing burnout.
Example: Accountants use AI for invoice processing, enabling deeper financial analysis.
Enhanced Productivity
Streamlined workflows and real-time tools improve efficiency.
Example: Customer service teams leverage AI for faster resolutions and better productivity.
D. New Revenue Streams
AI-Driven Offerings
Businesses create revenue through AI-powered products and services.
Example: Software companies offer AI-based marketing automation platforms.
Optimized Pricing
AI refines pricing strategies and predicts demand to maximize profits.
Example: Airlines dynamically adjust ticket prices with AI insights.
E. Competitive Advantage
First-Mover Edge
Early AI adopters establish industry leadership and unique capabilities.
Example: AI-powered chatbots gave early adopters superior customer service.
Agility and Responsiveness
AI enables real-time insights for faster, smarter decisions.
Example: Manufacturers use AI to identify bottlenecks and minimize downtime.
Risk Mitigation Costs: Protecting Your Investment
Implementing AI involves significant risks. To safeguard investments, maintain customer trust, and deploy AI ethically, businesses must account for these risk mitigation costs:
A. Data Privacy and Security
Protecting Sensitive Data
AI systems handle sensitive data like customer records. To prevent breaches, businesses must invest in encryption, access controls, and security audits.
Example: CRM systems using AI must comply with GDPR/CCPA to secure customer data.
Regulatory Compliance
Meeting industry-specific data privacy laws, like HIPAA in healthcare, demands legal expertise and compliance tools.
B. Addressing Bias and Ensuring Fairness
Reducing Algorithmic Bias
AI models can inherit biases from training data, necessitating diverse datasets and fairness-aware algorithms.
Example: AI hiring tools must avoid perpetuating gender or racial bias in decisions.
Ensuring Equitable Outcomes
Regular audits help maintain fairness in AI outcomes.
C. Explainability and Transparency
Improving Decision Understanding
Complex AI models need techniques like explainable AI (XAI) to clarify decision-making.
Example: Loan AI systems must explain decisions to customers for trust and regulatory compliance.
Building Trust
Transparent systems foster trust among users and stakeholders, vital in industries like finance and healthcare
D. Ethical Guidelines and Practices
Establishing Guidelines
Develop clear policies to address privacy, bias, job displacement, and misuse risks.
Example: Autonomous vehicles need ethics frameworks for unavoidable accident scenarios.
Promoting Responsibility
Provide AI ethics training and create oversight boards for compliance.
E. Continuous Monitoring and Auditing
Establishing Guidelines
Regular monitoring ensures systems perform as expected and adapt to evolving requirements.
Example: AI fraud detection systems require periodic updates to combat new fraud tactics.
Conclusion
As we’ve seen, the true cost of AI implementation goes well beyond the initial investment in technology. Hidden expenses such as data preparation, integration with legacy systems, and risk mitigation—including data privacy, algorithmic fairness, and ethical considerations—play a significant role. However, these costs are often balanced by the transformative benefits AI offers, such as improved customer experiences, actionable insights, employee satisfaction, new revenue streams, and a competitive edge.
To fully tap into AI’s potential, businesses must adopt a strategic approach that prioritizes long-term value. This includes careful planning to define clear objectives, investing in data quality, addressing risks proactively, equipping employees with the necessary skills, and continuously monitoring AI systems to ensure they deliver desired outcomes.
At Amzur, we help businesses navigate the complexities of AI implementation with services that cover strategic planning, data preparation, risk management, and employee training. Our team is committed to enabling seamless AI integration into your operations while maximizing ROI and minimizing risks.
To learn more, check out our on-demand webinar, where you’ll discover actionable strategies to successfully integrate AI into your business and enable its full potential.
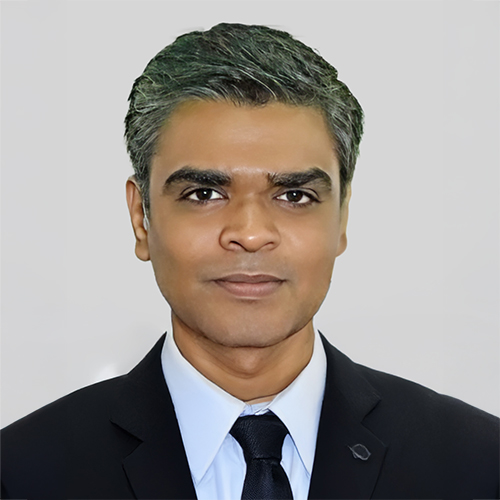
Director ATG & AI Practice